HL-LHC R&D
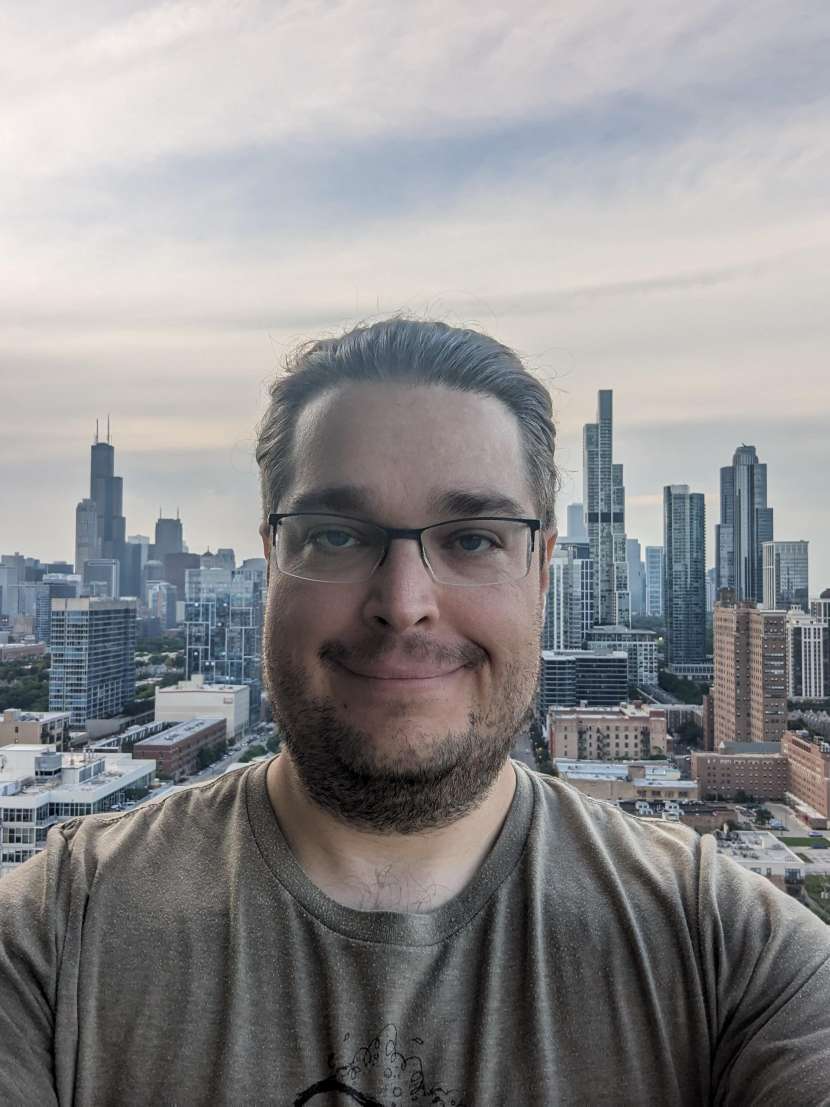
Lindsey Gray
Fermilab
Fermilab
HL-LHC R&D Co-Leader
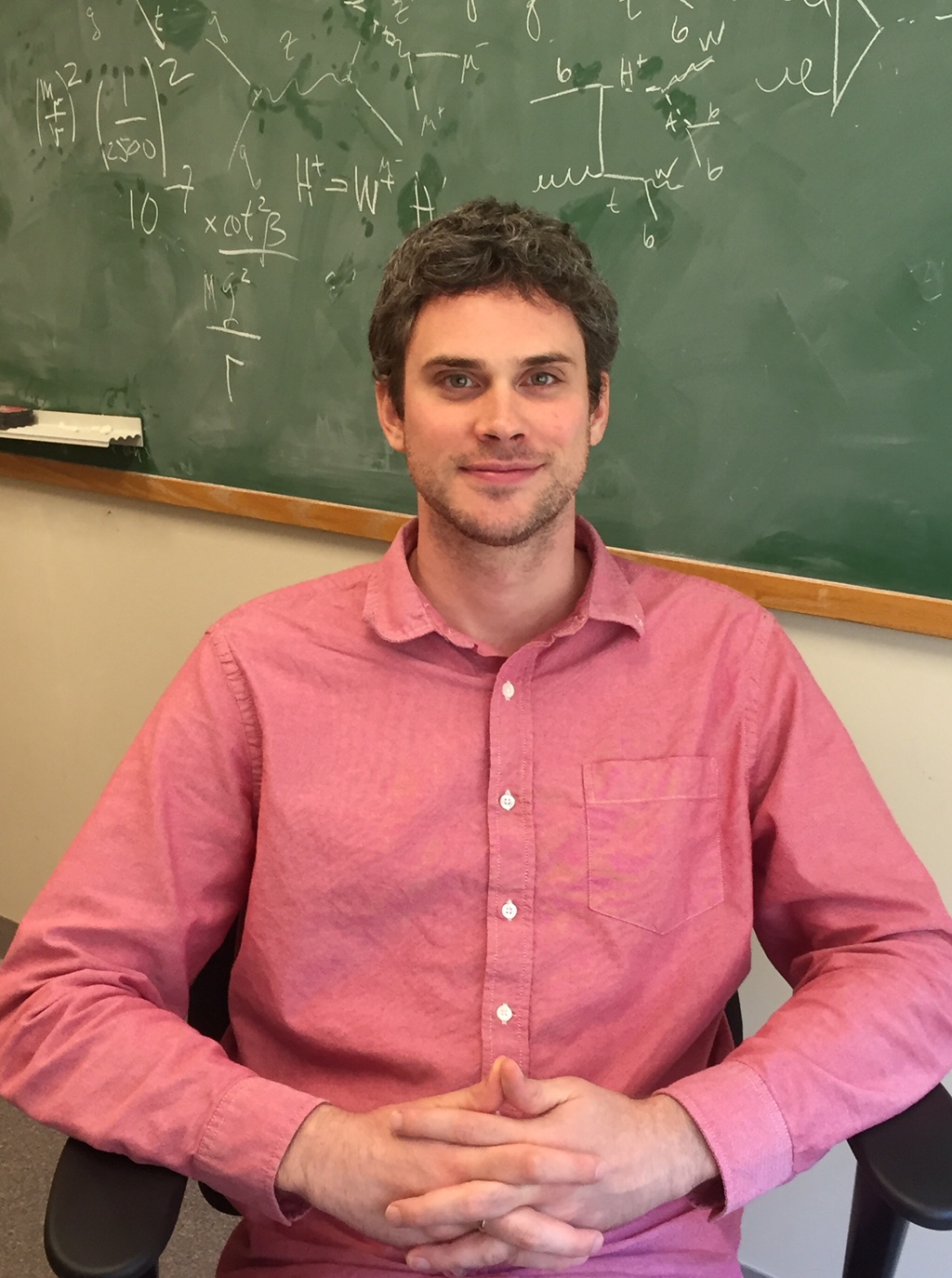
HL-LHC R&D Co-Leader
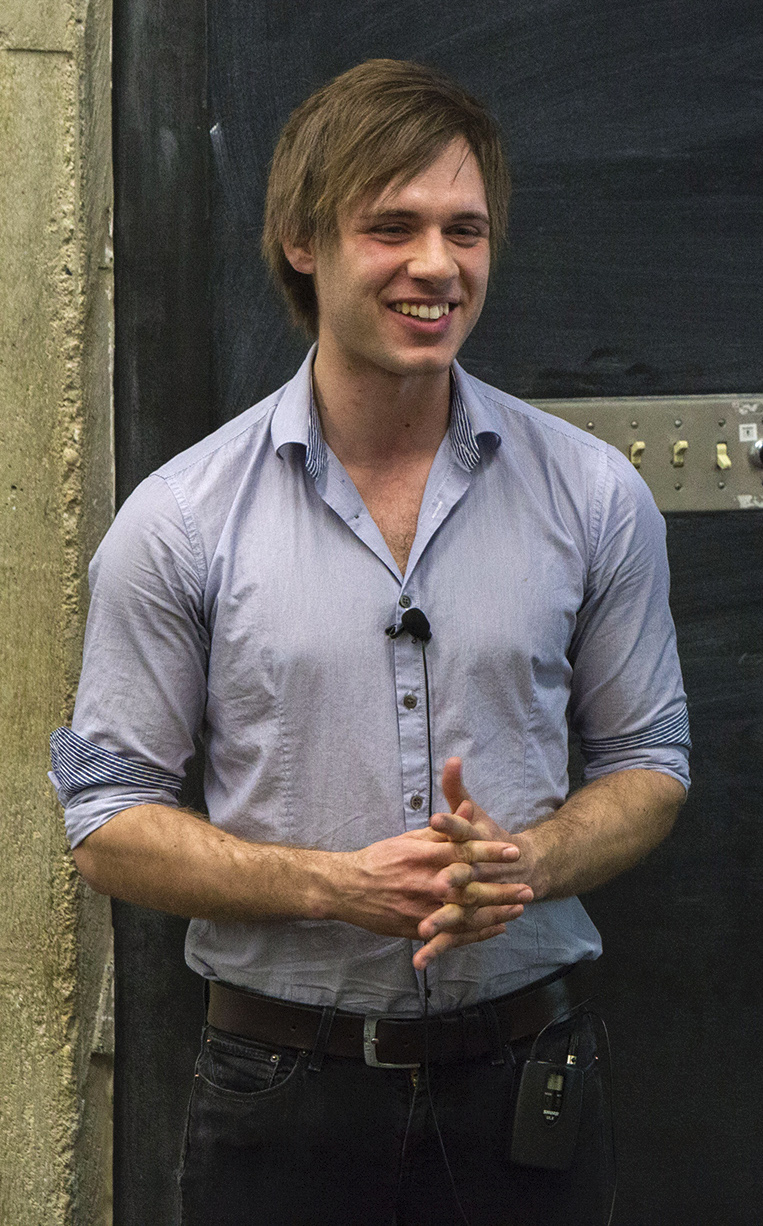
Matteo Cremonesi
Carnegie Mellon University
Carnegie Mellon University
HL-LHC R&D Analysis Systems Coordinator
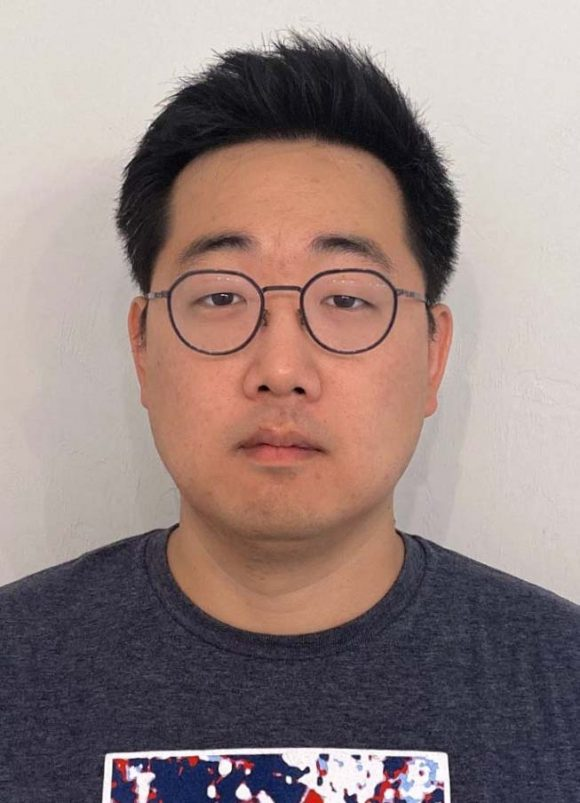
Philip Chang
University of Florida
University of Florida
HL-LHC R&D Algorithms Coordinator
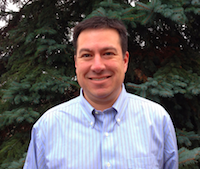
Computational Physicist
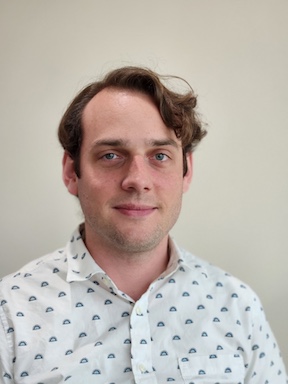
Nick Smith
Fermilab
Fermilab
U.S. CMS Fermilab Facility Architect
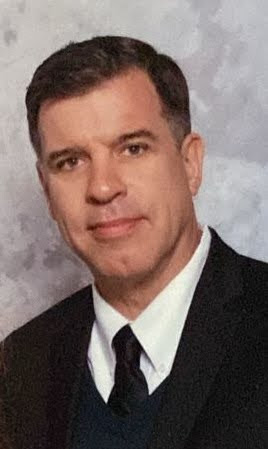
James Letts
UC San Diego
UC San Diego
U.S. CMS University Facility Architect
Overview
The HL-LHC area coordinates the centralized R&D effort for tackling the challenges in computing, data, and analysis during the HL-LHC era. We see the HL-LHC challenges as the following:
- Reduce the resources required (such as CPU and disk) by the HL-LHC physics program to levels seen as supportable.
- Enable analysis of much larger datasets as existing tools are not seen to scale up with the event count.
- Grow our pool of resources by more efficiently using owned resources, leveraging new resource types (accelerators), and using new resources such as HPC centers.
This R&D area is organized into three sub-areas:
- Analysis systems: developing new facilities and approaches for analysis during the HL-LHC era.
- Production infrastructure research: evolving the existing grid infrastructure and systems to meet the challenges of HL-LHC.
- Physics algorithms and tools: provide infrastructure and software to address the issues related to code performance in order to reduce computational needs for HL-LHC.
The area consists of software professionals and postdocs working on targeted year-long projects; there are significant collaborations with other projects and entities such as IRIS-HEP, Open Science Grid, HEP-CCE, ESNet, and SLATE.
Project Organization
The detailed area organization is:
- Analysis Systems: Develop tools and analysis systems for HEP that enable both innovation and the adoption of “industry standard” analytic techniques. Enable rapid interactive analysis of PB datasets.
- Tools for Advanced Analysis Provide interfaces and infrastructure to adapt HEP data in order to enable rapid analysis; projects include investments into columnar data such as Awkward Array.
- Analysis Facilities. Prototype and put into production the infrastructure required for a multi-user analysis facility exploiting the newly-developed analysis tools.
- Computing and Software Infrastructure: Explore, evaluate, prototype, and build the infrastructure necessary for HL-LHC computing.
- Storage: Evaluate storage technologies for performance; update data formats and data-handling for efficient use and rapid transfer.
- Provisioning of Compute Services: Simplify and automate the deployment of computing services through tools like Kubernetes.
- HPC Integration & Development: Develop workflow infrastructure to allow efficient use of LCF HPCs.
- Workflow Development: Research/prototype alternatives to bespoke CMS workflow management.
- AI/ML Infrastructure: Evaluate and construct methods of integrating AI training workflows for rapid development.
- Physics Algorithms: Provide infrastructure and software to address the issues related to code performance in order to reduce computational needs for HL-LHC.
- Adaptation for heterogeneous architectures: Convert or extend existing algorithms to run on accelerators.
- Algorithm Development: R&D into new algorithms, including those based on Machine Learning, that promise dramatic increases in processing speed.